Email Spam Machine Learning: Transforming Business Communication
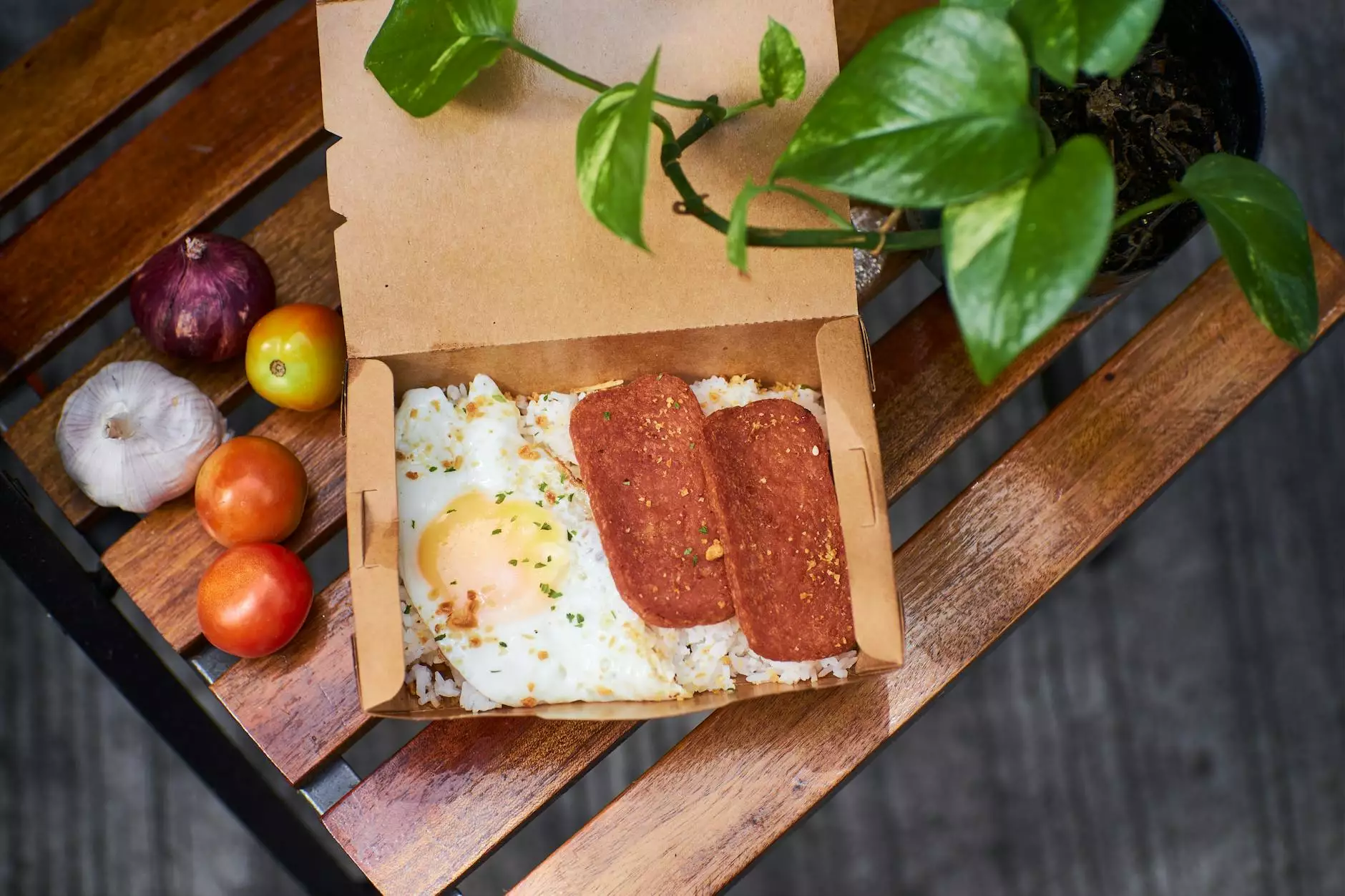
In today's digital age, email communication remains a cornerstone of business operations. However, with the rise of digital communication, the volume of email spam has also surged, leading to significant challenges for individuals and organizations alike. This article delves into how email spam machine learning has emerged as a powerful solution to combat unsolicited emails, improve productivity, and enhance overall business communication efficiency.
The Importance of Email Spam Management
Managing email spam is crucial for businesses for several reasons:
- Increased productivity: Employees spend valuable time sifting through spam emails, which can detract from their core responsibilities.
- Enhanced security: Spam emails are often a vector for phishing attacks and malware, posing a significant threat to organizational security.
- Improved communication: By filtering out unwanted emails, businesses can ensure that important communications are prioritized and not lost in a cluttered inbox.
Understanding Email Spam Machine Learning
Email spam machine learning involves the application of artificial intelligence (AI) and machine learning (ML) techniques to automate the detection and filtering of spam emails. By training algorithms on vast datasets of emails, businesses can significantly enhance their ability to identify unwanted messages accurately. Here’s a closer look at how this technology works:
How Machine Learning Works in Email Spam Filtering
At its core, email spam machine learning uses historical email data to train models that can classify incoming emails as either spam or not spam. The process typically involves the following steps:
- Data Collection: Large datasets of labeled emails (spam and legitimate) are gathered.
- Feature Extraction: Features such as keywords, phrases, and sender information are extracted from the emails to aid classification.
- Model Training: Machine learning algorithms, such as Support Vector Machines, Decision Trees, or Neural Networks, are trained using the dataset to recognize patterns associated with spam emails.
- Prediction: The trained model is then utilized to predict whether new incoming emails are spam based on the learned characteristics.
- Feedback Loop: Continuous learning through feedback from users helps refine the model, improving its accuracy over time.
Benefits of Implementing Email Spam Machine Learning
1. Enhanced Accuracy
One of the primary benefits of using machine learning for spam detection is its enhanced accuracy. Traditional rule-based filtering often struggles with new types of spam that don’t conform to known patterns. Machine learning models, however, continuously learn and adapt to new spam techniques, ensuring that they remain effective over time.
2. Reduction in False Positives
Early spam filters had a significant problem with false positives, where legitimate emails were incorrectly classified as spam. Modern machine learning approaches significantly reduce this issue, allowing organizations to maintain better communication channels without interruptions from legitimate contacts.
3. Automated Processes
With machine learning, businesses can implement automated spam filtering systems that require minimal human intervention. This automates the process of identifying and segregating spam emails, freeing up valuable employee time and resources.
4. Improved Security Posture
By accurately filtering out spam emails, machine learning systems help enhance the cybersecurity framework of a business. They reduce the risk of phishing attacks and malware infections that often come bundled with unsolicited emails.
Implementing Email Spam Machine Learning Solutions
For organizations looking to enhance their email security and filtering mechanisms, here are several steps to implement email spam machine learning solutions effectively:
1. Assess Your Current Email System
Evaluate your existing email infrastructure and determine the primary challenges you face regarding spam. This assessment will help you understand what solutions will be most effective.
2. Choose the Right Machine Learning Model
Different machine learning models are suitable for spam detection, including:
- Naive Bayes: Effective for its simplicity in probabilistic reasoning.
- Random Forest: Great for handling large datasets with complex features.
- Neural Networks: Especially beneficial for capturing non-linear relationships in data.
3. Train Your Model
Gather sufficient data to train your chosen model. Use a combination of spam and non-spam emails to build a robust training set. It’s crucial to ensure that the dataset is representative of the emails your organization typically receives.
4. Monitor and Refine
After implementing your model, continuously monitor its performance. Use feedback mechanisms to identify false positives and adapt the model accordingly to maintain its accuracy and effectiveness in spam detection.
Real-World Applications of Email Spam Machine Learning
Many organizations have successfully employed email spam machine learning technologies to streamline their operations. Here are a few examples:
- Gmail: Utilizes sophisticated machine learning algorithms to filter millions of spam emails every day, allowing users to enjoy a cleaner inbox.
- Microsoft Outlook: Implements machine learning to adaptively learn from user feedback and improve spam detection.
- Corporate Security Solutions: Various IT service providers use custom machine learning models tailored to the specific needs of their businesses to enhance email security.
Conclusion
As the digital landscape continues to evolve, the challenges posed by email spam are becoming increasingly sophisticated. Implementing email spam machine learning solutions provides organizations with a powerful tool to combat these challenges. By leveraging advanced algorithms and continuous learning capabilities, businesses can significantly enhance their email management practices, leading to improved productivity, security, and communication.
Understanding and adopting these machine learning solutions allows businesses to focus on their core operations while ensuring that their communication channels remain clear and effective. This not only safeguards their information security but also promotes a more efficient workplace, ultimately contributing to business success.